Introduction
In Nscale's latest technical deep dive, we explore a critical aspect of AI model optimisation: throughput benchmarking, performance tuning, and latency reduction using GEMM (General Matrix Multiplication) tuning.
Maximising the performance of GPU-accelerated tasks involves more than just raw speed. Optimising GEMM ensures efficient processing, higher throughput, and the ability to handle complex models and datasets effectively.
In this blog, we will explore the benchmarking of vLLM throughput across multiple models and delve into the significant impact of GEMM tuning. Powerful libraries such as rocBLAS (ROCm Basic Linear Algebra Subprograms) and hipBLASlt (Heterogeneous-Compute Interface for Portability, Basic Linear Algebra Subprograms) are instrumental in this process.
These libraries provide optimised implementations of GEMM operations along with a range of tuning parameters, allowing developers to fine-tune their applications and unlock the full potential of their underlying hardware, ultimately maximising vLLM performance.
What is GEMM Tuning?
GEMM tuning is a powerful technique for enhancing the performance of matrix-multiplication operations. This process includes selecting the most appropriate algorithm based on factors such as memory, cache, and compute capabilities."
By fine-tuning parameters and selecting optimal algorithms, we ensure the GEMM operation maximises efficiency in using available computing resources. This translates to significant speed improvements for AI and machine learning models.
Key Aspects of GEMM Tuning
Algorithm Selection:
When tuning GEMM operations, several key aspects must be considered to optimise performance. The first step is algorithm selection, which involves choosing the most efficient method for matrix multiplication based on the hardware's characteristics.
One common approach is the blocked algorithm, where input matrices are divided into smaller blocks. Matrix multiplication is then performed on these blocks, which enhances cache utilisation and parallelism by working on smaller data sets.
Another method is the tiled algorithm, similar to the blocked approach but with a greater emphasis on data locality and minimising memory access. This method breaks the matrix into smaller tiles and performs multiplication on these tiles, making it ideal for efficient use of shared memory.
For GPUs, parallel matrix multiplication algorithms are essential as they exploit parallelism inherent in GPU architectures. These algorithms utilise multiple threads or cores to compute matrix elements concurrently.
The choice between blocked, tiled, or other variants depends on factors such as matrix size, memory hierarchy, and the specific libraries in use on AMD GPUs and accelerators. Tools like rocBLAS (where BLAS stands for Basic Linear Algebra Subprograms) have built-in optimisations that automatically select the most appropriate algorithm based on these factors.
Parameter Adjustments:
Adjusting parameters such as block size or tile size to optimise performance. These adjustments help to align the operation with the hardware's architecture.
Kernel Configuration:
Configuring the kernels used in GEMM operations to optimise how computations are distributed across threads, registers, and memory. Proper kernel configuration minimises latency and maximises throughput by efficiently managing the workload distribution.
Benchmarking Prerequisites
For our benchmarking tests, we used a pre-configured Docker image and ensured the following software and libraries were installed:
- Docker Image
- ROCm 6.1.2
- Python 3.10.12
- PyTorch 2.5.0
- Triton 2.1.0
- Flash Attention 2.0.4
- rocBLAS 4.1.2
- hipBLASlt 0.8.0
- Rccl 2.18.6
- vLLM 0.5.0
We conducted our benchmarks using the benchmark_throughput.py script from the vLLM repository, which can be found here.
Benchmark Runs
Our benchmarking process involved two primary runs. Initially, we executed a benchmark using the out-of-the-box settings to establish a baseline performance measurement. This run provided insight into the standard performance metrics without applying specific optimisations.
Subsequently, we performed a second benchmark run after implementing GEMM tuning. This tuning process involved optimising the GEMM operations using rocBLAS and hipBLASit libraries, tailored to enhance computational efficiency and overall throughput, all of our benchmarks were conducted using the bf16 datatype.
Metrics Compared
Our analysis compared several key performance metrics between the two benchmark runs.
- Generation Speed (tokens per second): Allowed us to gauge the efficiency of token generation for both input and output processes.
- Requests per Second: Providing a clear indication of the system's ability to manage multiple concurrent requests effectively.
- Overall Throughput (tokens processed per second): Encapsulates the combined efficiency of generation speed and request handling, offering a comprehensive view of the system's performance under different configurations.
- Average Latency (seconds): Measuring the time taken to generate a response.
Settings for Benchmark Runs
We configured each benchmark run with the following settings:
- Input Prompt Length for Each Request: 256 tokens
- Output Length for Each Request: 256 tokens
- Tensor Parallel Size: 1 (utilising a single GPU, specifically the MI300X)
- Batch Sizes: 1, 2, and 4
Key Observations
Let’s delve into the notable advancements achieved through GEMM tuning of LLMs such as Llama, Mistral, Mixtral, and Falcon. We will analyse a series of graphs and data visualisations that elucidate the impact of Tuned GEMM on the performance and efficiency of these models.
Throughput/s:
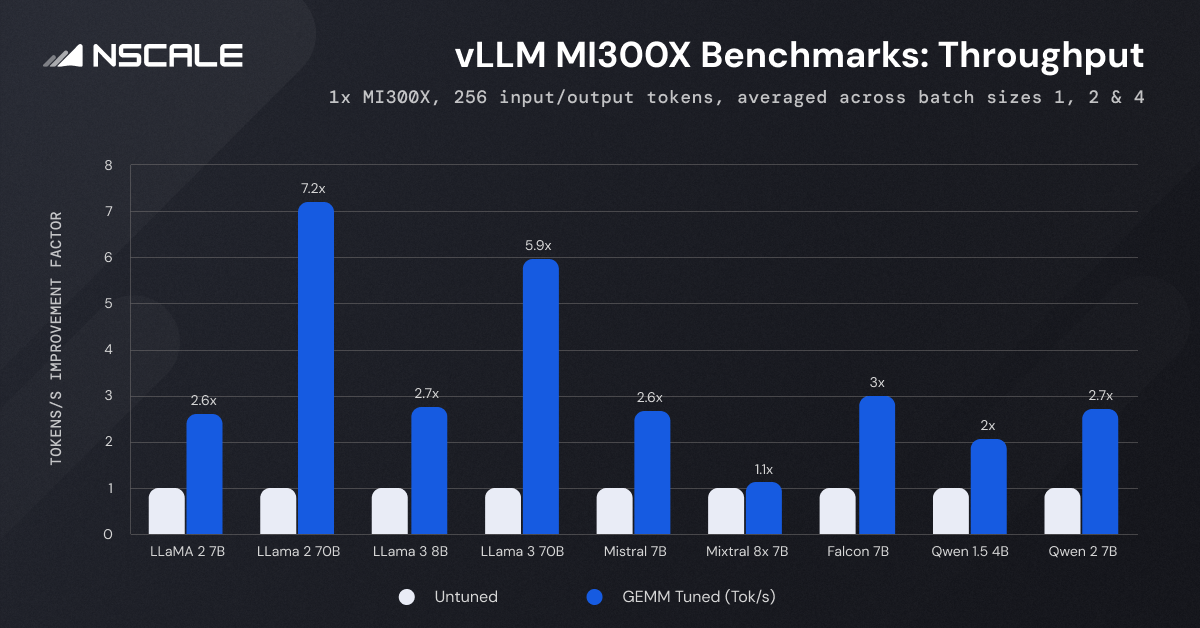
The graph shows a significant increase in generation speed when GeMM tuning is enabled
GEMM Tuning Impact: Enabling GEMM tuning increases throughput by up to 7.2x, as seen with the LLaMA-2-70B model.
Model Size: Larger models like LLaMA-2-70B and LLaMA-3-70B show the most significant improvements in throughput, with increases of 7.2x and 5.9x, respectively.
Batch Size: Higher batch sizes generally lead to greater throughput, amplified by GEMM tuning. For instance, throughput for the Falcon 7B model rises from 244.74 tokens/second at batch size 1 to 952.38 tokens/second at batch size 4 without GEMM tuning. With tuning, it climbs further to 2736.58 tokens/second.
Comparison Across Models: Among the models tested, LLaMA-2-70B and LLaMA-3-70B exhibit the highest throughput due to their complexity and size. Conversely, smaller models like Qwen 1.5 4B and Falcon 7B show relatively higher throughput, indicating more efficient processing for less complex models.
Latency:

The graph depicts the consistent reduction in latency achieved through GEMM tuning.
GEMM Tuning Impact: Latency reduces significantly across all models. For instance, latency for the LLaMA-2-7B model drops from 1.00 to 0.35 seconds. During testing, we observed that with GEMM tuning enabled, the latency of the LLaMA-2-7B model with a batch size of 1 dropped by 66.5% from 1.97 seconds to 0.66 seconds. This pattern holds true until a batch size of 4, highlighting the significant performance enhancement GEMM tuning offers.
Model Size: Larger models inherently exhibit higher latency. The LLaMA-2-70B model, for example, shows a latency of 1.00 seconds without GEMM tuning and 0.14 seconds with tuning enabled. In comparison, smaller models like LLaMA-2-7B show much lower latency under similar conditions. This trend is consistent across batch sizes, emphasising that model size directly impacts processing time.
Batch Size: While larger batch sizes typically increase latency, GEMM tuning mitigates this, maintaining lower latency. In our testing of the LLaMA-2-7B model without GEMM tuning, the latency rises from 1.97 seconds at batch size 1 to 2.11 seconds at batch size 4. With GEMM tuning enabled, the increase is from 0.66 seconds to 0.77 seconds. This suggests that while GEMM tuning mitigates the latency increase to some extent, processing larger batches naturally requires more computational effort and time.
Comparison Across Models: Models like Qwen 1.5 4B and Falcon 7B also show reduced latency, emphasising the effectiveness of GEMM tuning across different complexities.
Conclusion
Our comprehensive benchmarking study of AMD MI300X GPUs with GEMM tuning reveals improvements in both throughput and latency, with gains of up to 7.2x in specific models. By optimising GEMM operations using rocBLAS and hipBLASlt libraries, we significantly enhanced the performance and efficiency of various large language models, including LLaMA, Mistral, Mixtral, and Falcon.
Key findings from our benchmarks include:
- Throughput Improvements: Enabling GEMM tuning resulted in notable increases in throughput across all models. Larger models such as LLaMA-2-70B and LLaMA-3-70B demonstrated the highest improvements, benefiting significantly from the optimised operations.
- Latency Reductions: GEMM tuning consistently reduced latency across different batch sizes and models, with notable decreases observed in large and smaller models. This optimisation ensures quicker processing times, which is crucial for real-time AI applications.
- Batch Size Impact: While increasing batch size typically affects latency and throughput, GEMM tuning effectively mitigated these impacts, maintaining high-performance levels even with larger batch sizes.
These results underscore the critical role of GEMM tuning in maximising the capabilities of AI models on AMD GPUs. By leveraging advanced tuning techniques, developers can unlock the full potential of their hardware, ensuring efficient processing and superior performance for complex and demanding AI workloads.
Discover the complete and updated benchmark results by accessing our full table of results here. Stay informed with the latest benchmark results.